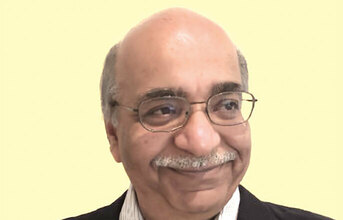
developed an autonomous guided robot for one of our European clients.
But often, even before adopting digital assistants, OEMs can be apprehensive of the effect this may have on human jobs. If anything, it elevates the role of human workers, allowing them to shift their attention to more meaningful tasks.
Moreover, digital assistants store vast amounts of information about product specifications and safety guidelines, giving quick access to information and streamlining operations.
LLM-powered digital assistants can also provide on-the-job training. New workers can receive stepby-step guidance from the assistant, allowing them to quickly get up to speed.
We too help our automotive clientele learn about nuances of new technology based on our experience across diverse industries and their needs and interests, ensuring that their business continues to scale and thrive.
How intuitive are LLMs in gauging road conditions and reducing road accidents?
Gauging road conditions, especially in a tropical country like India, can be tricky. However, large language models can process years of weather and vehicle movement data, correlate it to road conditions, providing accurate predictions that have the potential to be incredibly intuitive in gauging road conditions and potentially reducing road accidents.
It can process real-time data from various sensors, such as traffic signals, and weather stations, thereby predicting traffic congestion and weather patterns. This information can then be used to provide real-time warnings to drivers about potential hazards.
These models can also be integrated into the advanced driver assistance systems (ADAS) to provide real-time feedback to drivers. They can analyse a driver's behaviour and alert them if they are exhibiting signs of fatigue or distraction, helping reduce any road-safety related risks.
At Creative Synergies Group, we have trained our language models with a slew of data that we have processed internally, helping ensure accurate predictions.
Despite its capabilities, it's important to remember that LLMs are not a standalone solution; especially when it comes to road safety.
What are the drawbacks of LLMs application in the automotive industry?
A concerning drawback of language models is the amount of power it consumes. The carbon dioxideequivalent emissions produced by GPT-3 stood at 502 tonnes in 2022, the highest when compared to similarparameter trained models.
Several research firms and businesses are looking into green AI, which could soon become a possible solution. Until then, however, automotive businesses can benefit from analysing their use-case before deploying LLMs into their operations.
At Creative Synergies Group, we assess the needs of our clientele before leveraging any technological solutions, and the same holds true for LLMs. If it is not going to add any real value to their operations or products, we redirect them to the best suited technological solutions instead.
Where do Indian automotive companies stand in terms of tech adoption?
Over the past few years, Indian automotive companies have actively embracing innovation technologies into their products and processes. In 2021 alone, the Indian manufacturing sector spent USD $102 billion on manufacturing technology.
The shift towards electric mobility is an example of tech adoption in India. Several Indian automotive companies have introduced electric vehicles (EVs) and are investing heavily in EV technology.
However, it's worth noting that the pace of tech adoption can vary among different companies. While some are leading the charge in embracing new technologies, others might still be in the early stages of adoption.
Despite challenges, Indian automotive are growing focus on research and development, collaboration with technology partners, and government support for innovation.
With the ongoing tech maturity, how will the responsibility of LLMs in the automotive industry evolve in the next five to ten years?
There will always be a better use-case for something that is already in use today. We are currently in the initial stages of advanced AI development and as the technology matures, it will become capable of a lot more complex functions.
As LLMs become more ingrained in critical systems like autonomous vehicles, the demand for understanding their decisions will only grow. As a result, we can expect to see language models that are more transparent and interpretable, striking a balance between complexity and explainability.
Technology advances will lead to more power-efficient hardware and innovative algorithms, enabling LLMs to run more efficiently even on resource-constrained automotive systems.
As LLM technology becomes widespread, we can anticipate cost-effective solutions. The industry will find ways to integrate these language models more seamlessly into existing systems, making their adoption more accessible for automotive companies of all sizes.