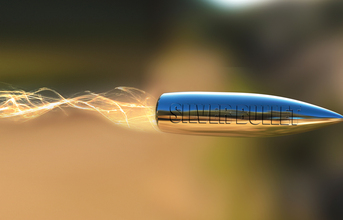
APO: The New Saviour?
With newer technologies of data processing, people found a "technology" solution to the MRP II problem. A good algorithm of optimisation, which can do a simultaneous check against multiple constraints, can provide answers faster than the sequential and batch process approach of MRP II technologies could.
The key selling point was the best of both worlds - optimal usage of capacity and other limiting conditions (material or tools) along with high on-time delivery. The capability to do fast on-line processing also made "what-if" analysis and rescheduling much easier than MRPII did. "On paper", one had found out the way to avoid overloads, right in the planning phase when order due dates were being set. It seemed as if everyone had finally got the elusive silver bullet!
However most of the implementations of APO failed to give the desired results - overloads happened in execution. The feature of frequent rescheduling actually did not help much. The plants which tried to do so had to stop it immediately because frequent rescheduling amplified a small uncertainty into chaos in the shop floor. In some cases, the schedules churned out by APO tools did not make intuitive sense to shop floor managers so they did not follow it.
Faulty Assumption of APO systems
APO was built in a lab without considering the "real world". The practical world has two problems, which make it difficult to define capacity accurately at any point of time. First, Variability is a way of life - there is no perfect plant without breakdowns, rejections, absenteeism, and even changing demand requests. And second is the changing product mix (capacity available can change based on product mix loaded on the plant at a point of time).
The combined effect of these two factors makes it difficult to precisely define capacity. Product mix changes impact capacity and so does variability in terms of worker skill, machine conditions and many other factors. It is nearly impossible to consider all possible factors that can accurately define capacity at a point of time.
Because of the above conditions, it is difficult for a computer schedule to match the intuition of a plant manager. A plant manager will always have more information (not considered by the computer) to arrive at a "better" decision. For example a dyeing department would want a specific colour sequence to maximise its output but the subsequent spinning department would want a conflicting sequence based on its need to produce a desired sequence of "count" of the yarn.
In the real world, the schedule given by a computer, which has globally considered both, may not be acceptable to either work centre manager. On a specific day, the dyeing manager may want to avoid making the difficult shade of colour (as per the schedule generated by the computer), because the most experienced person who can do the colour mixing without rejections for the "difficult" shade is absent.
At the same time, the spinning manager might want to avoid taking up a particular schedule because it is not productive to produce the specific yarn after breakdown maintenance. Such numerous considerations cannot be incorporated in the capacity definition because at times such conditions are also not "rigid" enough to be followed. Considering all such numerous conditions as rigid can de-rate the overall capacity of the plant.
The other problem originated from the very stated advantage of APO algorithms they can optimise under environment of multiple constraints. In an environment of dependencies (the way one schedules a particular work centre has an indirect impact on subsequent work centre), it is mathematically impossible to maximise the usage of multiple constraints to the full capacity.
At the same time, when there is variability in the system, namely shortages, rejections, breakdowns etc, there is a need to leave aside adequate buffers in all identified constraint to maintain stability in the system. Without adequate buffers, one would be forced to reschedule very frequently with even minor variation. When multiple work centers are rescheduled on every variation, de-synchronisation sets in feeding departments making the plant chaotic.
The waiting time can amplify many times over multiple work centers. Alternatively, if one wants to have a stable schedule from an APO in an environment of multiple constraints and seemingly conflicting objectives, the level of buffers required at multiple places would make the plant stable but reduce the output significantly.
APO investment ultimately resulted in lot of efforts and resources without any meaningful outcome. The plant performance in terms of on-time performance remained at the same level.
(article continued on next page)