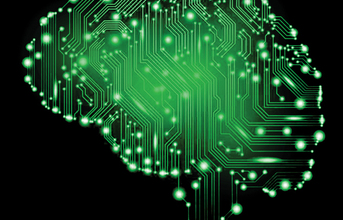
Machine learning technologies were used even as early as the year 2000, when the computer chip embedded in a truck's engine would adapt a varied algorithm to maximise its operating efficiency. For example, the truck being driven on plain road would have a different efficiency algorithm than when it is being driven on high-terrain. Auto-insurance premiums have been determined by collecting, monitoring and analysing the driver's behaviour by key functions performed by the driver through a remote sensor placed in the car. If the driver pressed his brakes too hard continuously, or continued to drive over the speed-limit, he was associated with high-risk attributes and was charged high premiums. This method of dynamic assessment of driver behaviour for charging premiums was considered an innovative as good driving skills could be rewarded with lesser premiums in the future.
The concept of machine learning is to configure mathematical algorithms in the computer so that it can pick up prominent key words or patterns from huge amounts of data with minimal human intervention. Today, machine learning technology has evolved to capture real-time sensor data from various parts of the machines and be able to predict, using statistical modelling, the exact time when a part may need to be replaced. Terabytes of data produced at manufacturing plants are impossible to analyse by the human eye alone, but on the other hand, hold important information on risk and efficiency. Machine learning technology coupled with Predictive Analytics can detect the likelihood of a machine part of requiring servicing or replacement ahead of time, thus minimising production downtime and production of defective products. The role of the analyst here is also important as he needs to ensure that the analysis conforms to business logic and decisions from it will be positive.
Warranty or service contracts are other areas of potential savings and optimisation using a combination of machine learning and predictive modelling. Warranty is provided to customers along with the product sold, and may contain a variety of plans of how the customer may be able to fix a broken part for a period of time, and extended warranties are also sold to mitigate the risk of big repair costs in the future. However, focus on warranty services has not been a priority for manufacturers, and as a result a yearly global cost of US$70 billion is spent in this area. Poor data quality, manual warranty or claims process that are disconnected with product issues, lack of visibility or insight due to absence of analysis and functional teams working in silos are some of the reasons for heightened risk for fraud and poor supplier recovery. Once the data streams are consolidated and distilled, insights on a variety of business areas can be discovered, for example, patterns of fraudulent claims, faulty parts from a particular dealer or supplier, or detection of a machine part that is vulnerable and require reengineering. If the likelihood of a part breaking down often every few months is high, then the warranty service could be aligned towards addressing it. This would benefit the company save on warranty claims cost by proactively fixing problems before it occurs; it would also increase extended warranty revenues and brand value.